A game changer: the rise of artificial intelligence
26 October 2021
Artificial intelligence is gradually penetrating the securities lending industry, with firms attempting to replicate human intelligence in automating and applying predictive analytics to their lending processes. Carmella Haswell reports
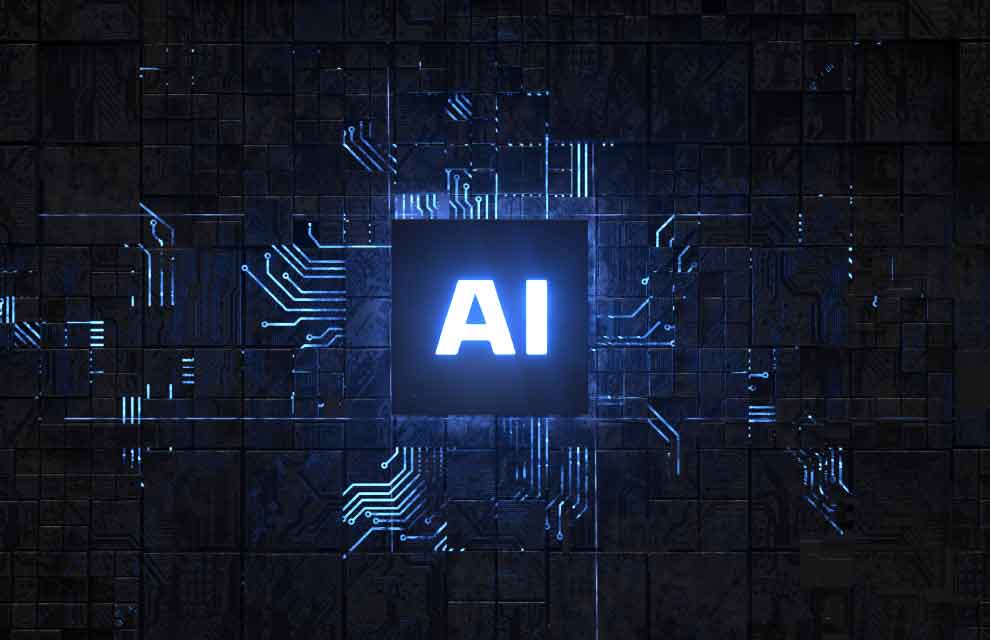
A rise in electronic trading platforms and the increasing availability of data has attracted the interest of a growing number of firms that have begun to progressively use artificial intelligence (AI) and machine learning (ML) in their trading and advisory activities, risk management and compliance functions.
The use of AI and ML could be a game changer in terms of providing better utilisation of data when it comes to how the industry makes decisions around inventory, says Matt Jones, global head of GM equities operations at Credit Suisse, at the DTCC CSDR Series 2021 panel in March.
Guidance from the International Organisation of Securities Commissions (IOSCO) reveals that the use of AI and ML-based technology may benefit market intermediaries, asset managers and investors, increasing the efficiency of existing processes, reducing the cost of investment services and freeing up resources for other activities.
Following its consultation report in June titled “The use of artificial intelligence and machine learning by market intermediaries and asset managers”, the IOSCO guidance also warned that this type of technology can create and amplify risks that could undermine financial market efficiency, harming consumers and other market participants.
As these technologies become more advanced, their popularity is expected to rise across the industry, requiring regulators to keep a watchful eye on how these are applied and coordinate efforts to address emerging risks.
Emergence
AI and ML encompasses a range of applications from chatbot assistants to fraud detection and task automation.
Across the globe, we have seen the emergence of firms developing technologies to enhance progress towards an efficient working space. US-based asset servicing specialist BNY Mellon set out to tackle settlement failures by leveraging Google Cloud’s data analytics, AI and ML technologies, to develop the ‘first-of-its-kind’ collateral management and liquidity solution.
From across the Atlantic, UK-based Delta Capita, a business and technology consultancy, adopts Luminance. The AI platform is being used to assist with its London Inter-bank Offered Rate (IBOR), Inter-bank Offered Rate and the Euro Inter-bank Offered Rate (EUROIBOR) transition projects.
The technology will help better understand risk exposure across contracts and mitigate the impact of the transition, according to Delta Capita. Luminance is able to learn from the interaction of the reviewer to generate additional insight.
Deutsche Börse-owned post-trade services provider Clearstream is collaborating, both internally and with clients, to find appropriate use cases for AI technology. The firm is investing in educating and upskilling its staff in AI and ML applications, as well developing the tool sets available to its data scientists to train algorithms, Graphics Processing Units (GPUs) and distributed computing.
Speaking to SFT, Priya Sharma, head of client connectivity and data at Clearstream, says: “We have recently launched a predictive and interactive penalties, inefficiencies and buy-in volumes (PIBIV) dashboard to support our clients with the upcoming settlement discipline regime. It identifies cash penalties, buy-in volumes and settlement efficiencies.” Over 20 institutions have signed up for it and, according to Sharma, there are many others that are interested. “We are also working on settlement predictions,” she says.
Today, clients submit settlement instructions without having clear indication of whether the settlement will happen on time or not, notes Sharma. Soon, Clearstream anticipates it will be able to predict the probability of settlement failure for up to two days in advance. “The AI has been trained and its performance has been quantified,” she says. “If the AI says the settlement will fail, today it is 80 per cent of the time correct. So, it’s doing very well.
“We are also working with one of our clients and analysing their collateral data to predict the blockage of an account before it is actually blocked and give an early warning. The next step now is to model predictive insufficient collateral triggers.”
Clearstream has incorporated the use of natural language processing (NLP) to extract data for corporate action events from incoming swift messages, creating a golden copy of transaction data that can be sent to clients.
Replication
Firms are evolving the way they operate, with AI and ML technologies being used to replicate human behaviour. It becomes more than just a simple piece of software that will assist in improving the efficiency of a service, but instead incorporates human intelligence to run those services automatically.
“You start getting to the aspects of a business process, where you’ve either tried to predict the future, or you’re trying to replicate human thought processes, particularly where those relate to client relationships,” says Martin Walker, head of product, securities finance and collateral management at Broadridge. “It’s when you get to those kinds of areas, then you start looking beyond just normal software tools that simply implement rules to looking at how we can replicate a human.”
Broadridge is developing AI technology to automate management of share recalls and fail prediction services. With the use of ML, the company is creating a model where large data sets are employed to predict whether trades are likely to fail or not.
For recalls management, a fundamental process in securities lending, the initial phase of their proof of concept has been around getting the right data that can be used to automatically trigger the recall process.
Speaking to SFT, Walker says: “Two of the biggest triggers for asking for your stock back are corporate actions and corporate voting. Corporate voting is particularly important in the age of ESG because it’s not just about making investments, it’s about being a good custodian of the assets.”
In managing this process, Walker explains that a firm would typically examine this initial set of data to identify the primary triggers for recalling stock. It would then analyse historical data indicating which lender firms are most likely to recall stock that they have out on loan. “This is where you can start applying machine learning to predict who’s going to recall you and in which situations,” says Walker.
When discussing how to strike a balance between creating an efficient system using AI technology and providing a human element that can make the best decisions to benefit their clients, Walker says there needs to be a focus on statistical relationships.
With advanced technology, it can produce the most efficient process or predict a better solution to certain problems — but a primary limitation with the software lies in its inability to contextualise the bigger picture. “You will never get rid of people because people have that bigger picture context. A system can come up with amazing answers, but it doesn’t really understand what a trade or a security is. People have the fundamental understandings of the core concepts,” adds Walker.
Adoption
Like Walker, Yannick Bierre, head of principal lending at BNP Paribas, indicates that machine learning is being selectively applied to deliver greater efficiency and insight to securities lending transactions. He explains: “We see NLP being adopted more widely to handle the growing demand flows. Transforming the information received into precise data and orders allows for a more efficient process.
“Machine learning or even deep learning are areas of interest as we have entered an era of data,” he says. “A number of companies already rely on these technologies. In finance, this could for instance result in AI generating pricing rules or dynamic reporting depending on the data it is treating.”
The driving force for the adoption of NLP, ML and AI by firms circles around the demands of both lenders and borrowers. Providing the most advanced solutions for clients is a priority for the majority of firms, but the needs of both lenders and borrowers may differ significantly.
According to Bierre, borrowers typically are aiming to improve daily workflows “through the provision of a quicker, more accurate and tailored programme of responses and greater availability through automating a number of step-by-step, added-value tasks”. For lenders, their priorities commonly focus on risk, utilisation of assets and transparency. “Automation allows for a more efficient process and avoids human errors, which means reduction of operational risks,” adds Bierre.
As one of a number of companies incorporating the use of this advanced technology, BNP Paribas has several developments underway for their securities lending services. Harnessing NLP technology, the multi-asset servicing company has launched locate scraper, an application that reads “stock locates” (Bloomberg, email) made by a borrower and converts them into requests. Consequently, it captures client’s demands, translating them into messages in their trading tool GLAD, proposing automated replies, trade booking and sending confirmations.
Bierre says: “We are also working on strengthening our digital structure to support further AI developments. Going forward we intend to improve our locate scraper and virtual trader further with the help of AI. And this is just the tip of the iceberg when it comes to AI developments we are considering, on a long-term horizon for securities lending.”
Evolution
Speaking to SFT, Sharma discusses the reasoning behind Clearstream’s involvement with AI and whether there is pressure from competitors to follow suit in this journey towards a techno-driven future.
“We are our own best competitors, but at the same time we all need to learn and evolve. There are a lot of new technologies that the industry is looking into today. We must make every effort to understand them and see how these technologies can help improve our processes,” says Sharma.
She continues: “Clients are our priority and we are diligently working with them in understanding how we can help create efficiencies and improve their processes along with our own. We have launched predictive solutions and are working on creating more predictive services to support our clients. Clearstream has been leading some of those efforts in the industry today.”
On a similar note, Broadridge’s Walker adds: “You don’t just automate everything because you can, you automate it because it makes economic sense.”
Technology has been the way forward for many industries and it is constantly evolving to create a more efficient future. But this techno-domination has triggered concerns about the social implications of replacing humans with machines.
Sharma says: “With new technologies, job losses are always a concern, but the volume is also increasing significantly. While we are able to automate the processes, we are able to increase scalability and quality. A second aspect is market volatility. AI is a probability-based solution, so you never know when the market changes and the probability crumbles.”
Sharma also identifies heavy energy consumption, required to drive some AI applications, as an ethical concern that the firm isn’t taking lightly. She says: “Sustainability is crucial to us when using new technology such as AI. We have created a strong data product development framework where we first prototype and optimise the success rate before using all the data.”
In conclusion, Sharma says that it is in the predictive space where future AI applications may deliver major benefit — where additional information is created to empower the decision-makers, instead of being reactive. “I would like to see AI being used not only in the financial industry but also in other industries to create a sustainable environment and improved health,” she concludes. “After all, it’s humans running the AI, not the other way around.”
The use of AI and ML could be a game changer in terms of providing better utilisation of data when it comes to how the industry makes decisions around inventory, says Matt Jones, global head of GM equities operations at Credit Suisse, at the DTCC CSDR Series 2021 panel in March.
Guidance from the International Organisation of Securities Commissions (IOSCO) reveals that the use of AI and ML-based technology may benefit market intermediaries, asset managers and investors, increasing the efficiency of existing processes, reducing the cost of investment services and freeing up resources for other activities.
Following its consultation report in June titled “The use of artificial intelligence and machine learning by market intermediaries and asset managers”, the IOSCO guidance also warned that this type of technology can create and amplify risks that could undermine financial market efficiency, harming consumers and other market participants.
As these technologies become more advanced, their popularity is expected to rise across the industry, requiring regulators to keep a watchful eye on how these are applied and coordinate efforts to address emerging risks.
Emergence
AI and ML encompasses a range of applications from chatbot assistants to fraud detection and task automation.
Across the globe, we have seen the emergence of firms developing technologies to enhance progress towards an efficient working space. US-based asset servicing specialist BNY Mellon set out to tackle settlement failures by leveraging Google Cloud’s data analytics, AI and ML technologies, to develop the ‘first-of-its-kind’ collateral management and liquidity solution.
From across the Atlantic, UK-based Delta Capita, a business and technology consultancy, adopts Luminance. The AI platform is being used to assist with its London Inter-bank Offered Rate (IBOR), Inter-bank Offered Rate and the Euro Inter-bank Offered Rate (EUROIBOR) transition projects.
The technology will help better understand risk exposure across contracts and mitigate the impact of the transition, according to Delta Capita. Luminance is able to learn from the interaction of the reviewer to generate additional insight.
Deutsche Börse-owned post-trade services provider Clearstream is collaborating, both internally and with clients, to find appropriate use cases for AI technology. The firm is investing in educating and upskilling its staff in AI and ML applications, as well developing the tool sets available to its data scientists to train algorithms, Graphics Processing Units (GPUs) and distributed computing.
Speaking to SFT, Priya Sharma, head of client connectivity and data at Clearstream, says: “We have recently launched a predictive and interactive penalties, inefficiencies and buy-in volumes (PIBIV) dashboard to support our clients with the upcoming settlement discipline regime. It identifies cash penalties, buy-in volumes and settlement efficiencies.” Over 20 institutions have signed up for it and, according to Sharma, there are many others that are interested. “We are also working on settlement predictions,” she says.
Today, clients submit settlement instructions without having clear indication of whether the settlement will happen on time or not, notes Sharma. Soon, Clearstream anticipates it will be able to predict the probability of settlement failure for up to two days in advance. “The AI has been trained and its performance has been quantified,” she says. “If the AI says the settlement will fail, today it is 80 per cent of the time correct. So, it’s doing very well.
“We are also working with one of our clients and analysing their collateral data to predict the blockage of an account before it is actually blocked and give an early warning. The next step now is to model predictive insufficient collateral triggers.”
Clearstream has incorporated the use of natural language processing (NLP) to extract data for corporate action events from incoming swift messages, creating a golden copy of transaction data that can be sent to clients.
Replication
Firms are evolving the way they operate, with AI and ML technologies being used to replicate human behaviour. It becomes more than just a simple piece of software that will assist in improving the efficiency of a service, but instead incorporates human intelligence to run those services automatically.
“You start getting to the aspects of a business process, where you’ve either tried to predict the future, or you’re trying to replicate human thought processes, particularly where those relate to client relationships,” says Martin Walker, head of product, securities finance and collateral management at Broadridge. “It’s when you get to those kinds of areas, then you start looking beyond just normal software tools that simply implement rules to looking at how we can replicate a human.”
Broadridge is developing AI technology to automate management of share recalls and fail prediction services. With the use of ML, the company is creating a model where large data sets are employed to predict whether trades are likely to fail or not.
For recalls management, a fundamental process in securities lending, the initial phase of their proof of concept has been around getting the right data that can be used to automatically trigger the recall process.
Speaking to SFT, Walker says: “Two of the biggest triggers for asking for your stock back are corporate actions and corporate voting. Corporate voting is particularly important in the age of ESG because it’s not just about making investments, it’s about being a good custodian of the assets.”
In managing this process, Walker explains that a firm would typically examine this initial set of data to identify the primary triggers for recalling stock. It would then analyse historical data indicating which lender firms are most likely to recall stock that they have out on loan. “This is where you can start applying machine learning to predict who’s going to recall you and in which situations,” says Walker.
When discussing how to strike a balance between creating an efficient system using AI technology and providing a human element that can make the best decisions to benefit their clients, Walker says there needs to be a focus on statistical relationships.
With advanced technology, it can produce the most efficient process or predict a better solution to certain problems — but a primary limitation with the software lies in its inability to contextualise the bigger picture. “You will never get rid of people because people have that bigger picture context. A system can come up with amazing answers, but it doesn’t really understand what a trade or a security is. People have the fundamental understandings of the core concepts,” adds Walker.
Adoption
Like Walker, Yannick Bierre, head of principal lending at BNP Paribas, indicates that machine learning is being selectively applied to deliver greater efficiency and insight to securities lending transactions. He explains: “We see NLP being adopted more widely to handle the growing demand flows. Transforming the information received into precise data and orders allows for a more efficient process.
“Machine learning or even deep learning are areas of interest as we have entered an era of data,” he says. “A number of companies already rely on these technologies. In finance, this could for instance result in AI generating pricing rules or dynamic reporting depending on the data it is treating.”
The driving force for the adoption of NLP, ML and AI by firms circles around the demands of both lenders and borrowers. Providing the most advanced solutions for clients is a priority for the majority of firms, but the needs of both lenders and borrowers may differ significantly.
According to Bierre, borrowers typically are aiming to improve daily workflows “through the provision of a quicker, more accurate and tailored programme of responses and greater availability through automating a number of step-by-step, added-value tasks”. For lenders, their priorities commonly focus on risk, utilisation of assets and transparency. “Automation allows for a more efficient process and avoids human errors, which means reduction of operational risks,” adds Bierre.
As one of a number of companies incorporating the use of this advanced technology, BNP Paribas has several developments underway for their securities lending services. Harnessing NLP technology, the multi-asset servicing company has launched locate scraper, an application that reads “stock locates” (Bloomberg, email) made by a borrower and converts them into requests. Consequently, it captures client’s demands, translating them into messages in their trading tool GLAD, proposing automated replies, trade booking and sending confirmations.
Bierre says: “We are also working on strengthening our digital structure to support further AI developments. Going forward we intend to improve our locate scraper and virtual trader further with the help of AI. And this is just the tip of the iceberg when it comes to AI developments we are considering, on a long-term horizon for securities lending.”
Evolution
Speaking to SFT, Sharma discusses the reasoning behind Clearstream’s involvement with AI and whether there is pressure from competitors to follow suit in this journey towards a techno-driven future.
“We are our own best competitors, but at the same time we all need to learn and evolve. There are a lot of new technologies that the industry is looking into today. We must make every effort to understand them and see how these technologies can help improve our processes,” says Sharma.
She continues: “Clients are our priority and we are diligently working with them in understanding how we can help create efficiencies and improve their processes along with our own. We have launched predictive solutions and are working on creating more predictive services to support our clients. Clearstream has been leading some of those efforts in the industry today.”
On a similar note, Broadridge’s Walker adds: “You don’t just automate everything because you can, you automate it because it makes economic sense.”
Technology has been the way forward for many industries and it is constantly evolving to create a more efficient future. But this techno-domination has triggered concerns about the social implications of replacing humans with machines.
Sharma says: “With new technologies, job losses are always a concern, but the volume is also increasing significantly. While we are able to automate the processes, we are able to increase scalability and quality. A second aspect is market volatility. AI is a probability-based solution, so you never know when the market changes and the probability crumbles.”
Sharma also identifies heavy energy consumption, required to drive some AI applications, as an ethical concern that the firm isn’t taking lightly. She says: “Sustainability is crucial to us when using new technology such as AI. We have created a strong data product development framework where we first prototype and optimise the success rate before using all the data.”
In conclusion, Sharma says that it is in the predictive space where future AI applications may deliver major benefit — where additional information is created to empower the decision-makers, instead of being reactive. “I would like to see AI being used not only in the financial industry but also in other industries to create a sustainable environment and improved health,” she concludes. “After all, it’s humans running the AI, not the other way around.”
NO FEE, NO RISK
100% ON RETURNS If you invest in only one securities finance news source this year, make sure it is your free subscription to Securities Finance Times
100% ON RETURNS If you invest in only one securities finance news source this year, make sure it is your free subscription to Securities Finance Times
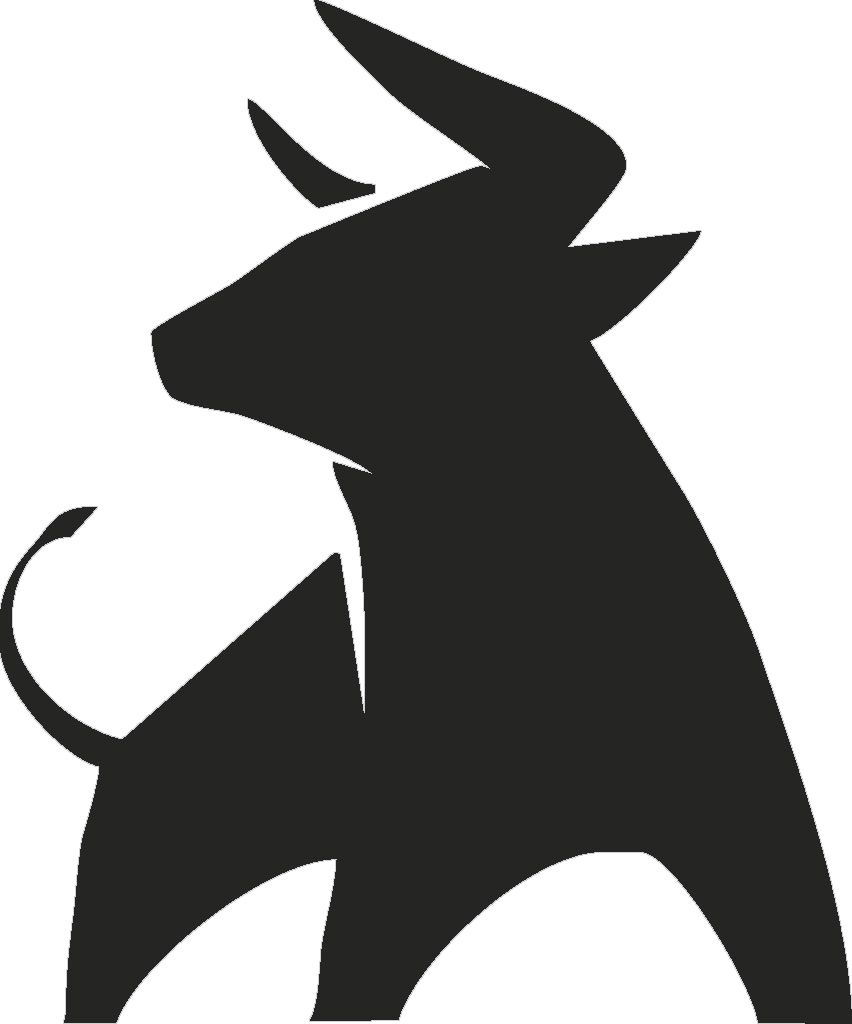