A winning combination
02 October 2018
Aurélien Manson of CACEIS explains why securities lending paired with machine learning is a winning combination
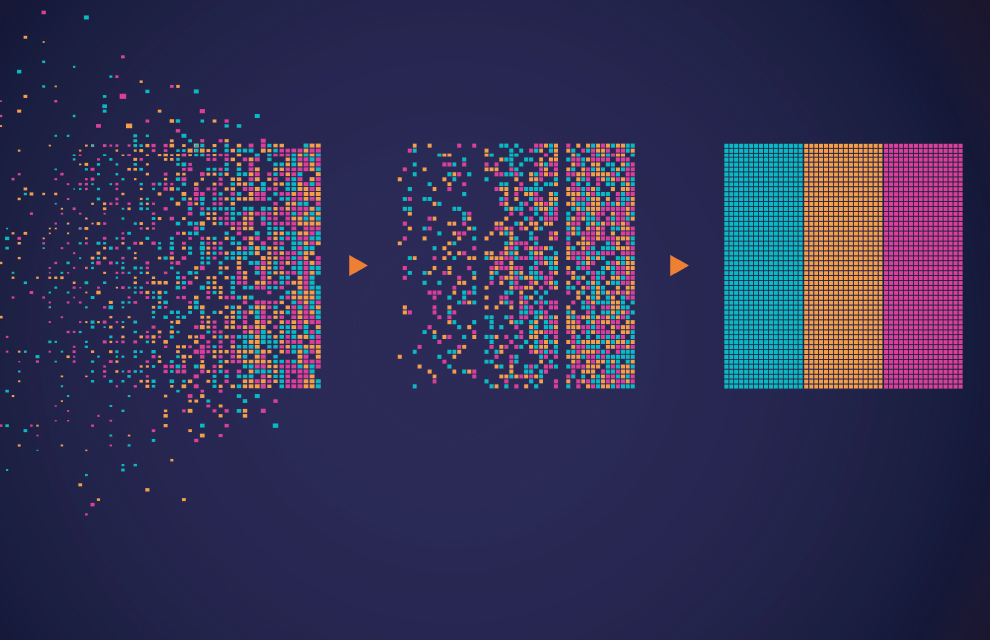
To reduce the workload of securities lending desks and enhance client returns, asset servicing companies are employing sophisticated machine learning algorithms developed in-house. Such companies offer securities lending programmes to pension funds, insurers and asset managers that use sophisticated artificial intelligence software to raise efficiency in pricing corporate bond loans.
The self-optimising algorithms at the heart of this artificial intelligence (AI) technology improve themselves with each iteration especially when they are able to work on large data sets, and lending programmes equipped with such technology are already seeing enhanced returns and reduced risks, which help bring them into line with leading players in the market.
For securities lending operations, machine learning and AI will be a key part of the business going forward, not just in the securities lending space but across all front and back office operations. Straight-through processing (STP) automation was the industry’s buzz phrase years ago, and indeed automation rates are still an important efficiency metric, however, machine learning has little in common with the former. Machine learning is a process based on powerful algorithms that constantly improve themselves in order to achieve better results each time. There are many everyday examples of machine learning in action, from natural language processing in speech recognition to YouTube’s video recommendation engine.
For the asset manager, the only noticeable impacts on the day-to-day business of machine learning in securities lending are faster responses, increased reliability, lower risk and improved remuneration opportunities. Many asset managers are seeing the benefits of leveraging massive datasets, computing power and machine learning software to optimise their trading and enhance yields.
Machine learning is having a positive impact on remuneration opportunities within securities lending and is also helping to reduce rates of operational error risk. In the current environment, where revenues are under pressure due to increasingly restrictive regulations, the use of machine learning to raise the efficiency of the standard securities lending process represents an important business development opportunity.
Securities lending desks receive large volumes of email every day quoting many underlying holdings and to quote a competitive price, it is necessary to check over 20 features for each asset. Companies need to think smarter rather than just increase manpower for such tasks. Automation and STP can only take you so far, but true machine learning is providing a revolutionary solution.
Leveraging innovation within a mature product category is a central part of asset servicing providers’ strategies. Asset servicing firm must offer up-to-date technology to clients. New technologies such as machine learning enable businesses to allocate human resources in a more efficient way, so it’s a win/win trade. Human resources focus on the added-value tasks, and the more repetitive and robotic tasks can be automated and then incrementally improved via machine learning techniques.
Most companies today are looking at how automation can improve their internal processes and many find that a high percentage of low-value administrative tasks traditionally performed by humans can be taken on by AI software. However, human traders remain essential for managing parameters further up in the process and still out-perform AI in soft data situations such as news analysis, profit warnings, bad/missing data or features not taken into account by the algorithms.
By reducing the role of humans in the process, and replacing them with well-designed AI software, error risk sees a corresponding reduction. A key role for custodians is to have visibility on and restitution capabilities for client assets. Machine learning algorithms make it possible to track each asset to determine how much can safely be lent/or needs to be borrowed to rebuild a buffer.
Machine learning algorithms: a look behind the scenes
When emails from counterparties arrive, algorithms scan them for information on asset types and quantities. Next, they automatically poll a database of lendable assets, verify that the trade meets the rules in terms of tradable size, risk limits, liquidity risk and so on, then send an email response directly to the counterparty.
Most machine learning algorithms don’t have free reign in this context and are solely used to initiate the negotiation, however, advances will enable it to handle progressively more, from processing incoming emails all the way to the final booking, with human intervention only when necessary for client relationship matters.
In technical terms, securities lending desks must completely model a corporate bond within their systems, using more than 36 variables to classify the bond by three features: the level, the sensitivity to the utilisation rate, and the convexity. Polynomial regressions and trees are the main analyses used to perfect the model, which is performed on an extensive lendable assets directory to create a vast reference database.
To understand how the algorithm operates, imagine lendable assets are coordinates within a 3D space and the three axes, x, y and z correspond to the level, the sensitivity and the convexity. When a new bond needs to be priced, the technology models the bond calculates where its coordinates place it, then look around that point for the nearest neighbours. Using a k-NN algorithm, where k is the integer, you can, for example, look at the three ‘nearest neighbours’, and even weight them according to their proximity and provide a very accurate figure with the most efficient lending level.
It’s certainly a very technical topic, nevertheless, we have the award-winning expertise within CACEIS, and are also strengthening our collaboration with Crédit Agricole’s DataLab department, which centralises group-wide efforts to leverage innovative fintech solutions. Our clients will benefit greatly from the increases in efficiency, speed and returns that our investments into technology seek to provide
The self-optimising algorithms at the heart of this artificial intelligence (AI) technology improve themselves with each iteration especially when they are able to work on large data sets, and lending programmes equipped with such technology are already seeing enhanced returns and reduced risks, which help bring them into line with leading players in the market.
For securities lending operations, machine learning and AI will be a key part of the business going forward, not just in the securities lending space but across all front and back office operations. Straight-through processing (STP) automation was the industry’s buzz phrase years ago, and indeed automation rates are still an important efficiency metric, however, machine learning has little in common with the former. Machine learning is a process based on powerful algorithms that constantly improve themselves in order to achieve better results each time. There are many everyday examples of machine learning in action, from natural language processing in speech recognition to YouTube’s video recommendation engine.
For the asset manager, the only noticeable impacts on the day-to-day business of machine learning in securities lending are faster responses, increased reliability, lower risk and improved remuneration opportunities. Many asset managers are seeing the benefits of leveraging massive datasets, computing power and machine learning software to optimise their trading and enhance yields.
Machine learning is having a positive impact on remuneration opportunities within securities lending and is also helping to reduce rates of operational error risk. In the current environment, where revenues are under pressure due to increasingly restrictive regulations, the use of machine learning to raise the efficiency of the standard securities lending process represents an important business development opportunity.
Securities lending desks receive large volumes of email every day quoting many underlying holdings and to quote a competitive price, it is necessary to check over 20 features for each asset. Companies need to think smarter rather than just increase manpower for such tasks. Automation and STP can only take you so far, but true machine learning is providing a revolutionary solution.
Leveraging innovation within a mature product category is a central part of asset servicing providers’ strategies. Asset servicing firm must offer up-to-date technology to clients. New technologies such as machine learning enable businesses to allocate human resources in a more efficient way, so it’s a win/win trade. Human resources focus on the added-value tasks, and the more repetitive and robotic tasks can be automated and then incrementally improved via machine learning techniques.
Most companies today are looking at how automation can improve their internal processes and many find that a high percentage of low-value administrative tasks traditionally performed by humans can be taken on by AI software. However, human traders remain essential for managing parameters further up in the process and still out-perform AI in soft data situations such as news analysis, profit warnings, bad/missing data or features not taken into account by the algorithms.
By reducing the role of humans in the process, and replacing them with well-designed AI software, error risk sees a corresponding reduction. A key role for custodians is to have visibility on and restitution capabilities for client assets. Machine learning algorithms make it possible to track each asset to determine how much can safely be lent/or needs to be borrowed to rebuild a buffer.
Machine learning algorithms: a look behind the scenes
When emails from counterparties arrive, algorithms scan them for information on asset types and quantities. Next, they automatically poll a database of lendable assets, verify that the trade meets the rules in terms of tradable size, risk limits, liquidity risk and so on, then send an email response directly to the counterparty.
Most machine learning algorithms don’t have free reign in this context and are solely used to initiate the negotiation, however, advances will enable it to handle progressively more, from processing incoming emails all the way to the final booking, with human intervention only when necessary for client relationship matters.
In technical terms, securities lending desks must completely model a corporate bond within their systems, using more than 36 variables to classify the bond by three features: the level, the sensitivity to the utilisation rate, and the convexity. Polynomial regressions and trees are the main analyses used to perfect the model, which is performed on an extensive lendable assets directory to create a vast reference database.
To understand how the algorithm operates, imagine lendable assets are coordinates within a 3D space and the three axes, x, y and z correspond to the level, the sensitivity and the convexity. When a new bond needs to be priced, the technology models the bond calculates where its coordinates place it, then look around that point for the nearest neighbours. Using a k-NN algorithm, where k is the integer, you can, for example, look at the three ‘nearest neighbours’, and even weight them according to their proximity and provide a very accurate figure with the most efficient lending level.
It’s certainly a very technical topic, nevertheless, we have the award-winning expertise within CACEIS, and are also strengthening our collaboration with Crédit Agricole’s DataLab department, which centralises group-wide efforts to leverage innovative fintech solutions. Our clients will benefit greatly from the increases in efficiency, speed and returns that our investments into technology seek to provide
NO FEE, NO RISK
100% ON RETURNS If you invest in only one securities finance news source this year, make sure it is your free subscription to Securities Finance Times
100% ON RETURNS If you invest in only one securities finance news source this year, make sure it is your free subscription to Securities Finance Times
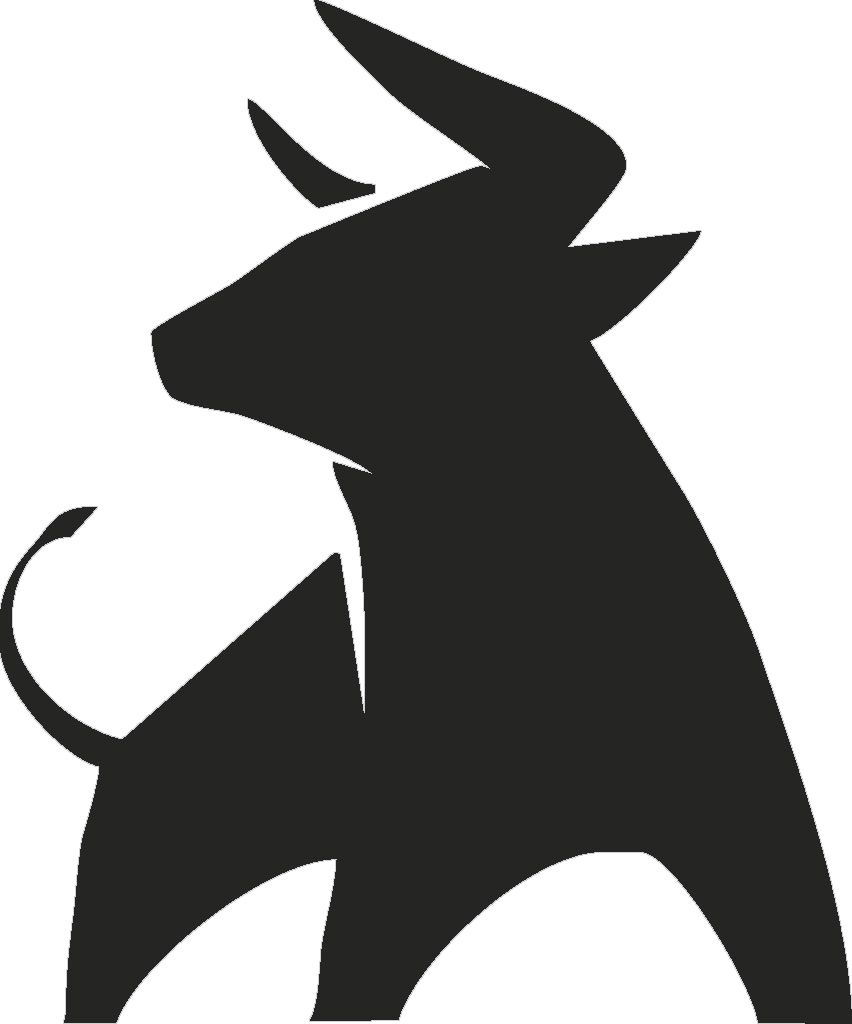