Machine learning in equity markets
08 January 2019
Stephanie Lo and Jian Wu of State Street discuss the intersection of machine learning applications and equity markets in portfolio management, exploring the potential market segmentation that will arise
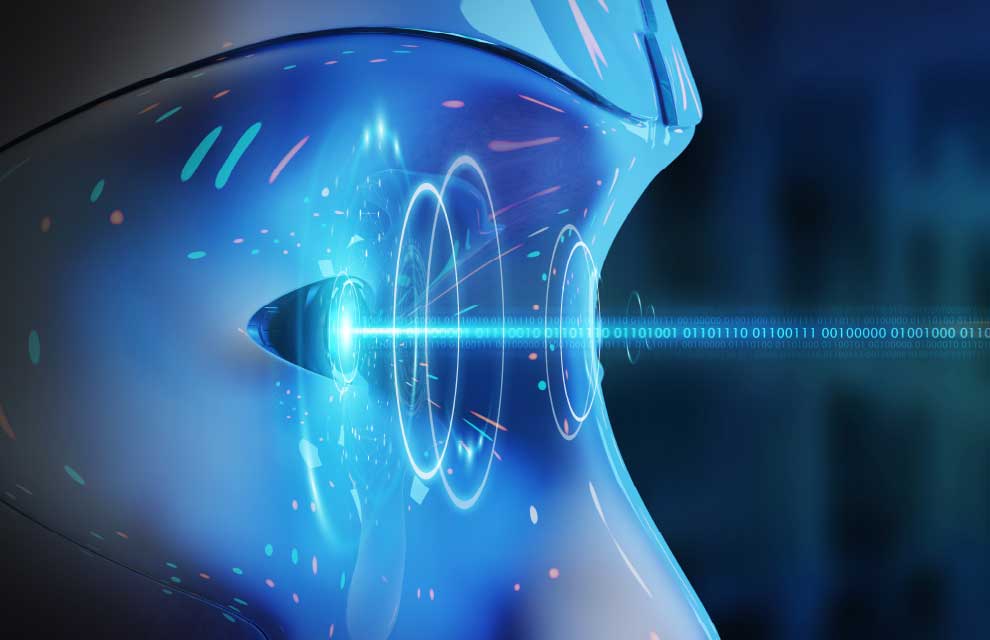
Artificial intelligence (AI) and machine learning (ML) are poised to change the way financial institutions operate. Yet the transformation will not impact all market players equally. In this piece, we examine the intersection of ML applications and equity markets in portfolio management and explore the potential market segmentation that will arise due to different adoption strategies of ML driven by each players’ mandates and goals.
The potential use cases for ML in portfolio management are broad, and in our view, likely segmented across different types of portfolio managers, with some representative considerations shown in figure one.
Factors driving resistance to machine learning in institutional investors
For most institutional investors, ML may be used in the research stages of portfolio construction, but are unlikely to enter the decision-making function. This is driven by several factors:
Case study
State Street Global Advisors has introduced three exchange-traded funds (ETFs) built with proprietary index methodologies developed by Kensho Technologies. The ETFs track companies that provide AI-relevant products and services; natural language processing (NLP) is used to parse through regulatory filings to understand company exposure to AI technologies.
Matthew Bartolini, head of SPDR Americas research at State Street Global Advisors, said: “The indices are constructed themselves using the very advanced technologies that the ETFs are trying to invest in.”
He added: “The ETFs are built around the fact that algorithms can dissect information in a very systematic way, processing data in 45 seconds that would take a human a full day to understand. In this case, AI is doing exactly what an investor would expect an intelligent human would do—while being highly efficient.”
Opportunities in ML for quant-driven hedge funds
Equities-focused hedge fund usage of ML has been substantial. One estimate is that pure ML players have about $10 billion in assets under management; this number would not include “commingled” strategies, in which ML methods are used to inform trades but there is still some discretion involved. Some drivers include:
Outlook for ML in beyond equities
In this piece, we have focused on ML in equity markets. While equity managers have taken some time to embrace ML technologies, it appears that interest is nearing the steep part of the curve, with usage expanding fairly rapidly. Looking at other parts of finance, we see multiple opportunities for ML:
As with all new technologies, the adoption curve for ML may be steep. As storage becomes cheaper, calculations become faster (for example, via graphics processing units, field programmable gate arrays, quantum computing, and so on), and data sources become increasingly diverse—and as ML becomes part of a standard academic curriculum—we anticipate that ML will become more commonplace in financial analysis. We are already starting to see more widespread adoption of ML in equity trading, and anticipate other financial markets—such as corporate bonds and treasuries—to follow. Until that future state, early adopters may benefit from the lack of widespread adoption to find alpha in differentiated strategies.
Figure one: Potential use case of machine learning equity inputs/factors, by group
The potential use cases for ML in portfolio management are broad, and in our view, likely segmented across different types of portfolio managers, with some representative considerations shown in figure one.
Factors driving resistance to machine learning in institutional investors
For most institutional investors, ML may be used in the research stages of portfolio construction, but are unlikely to enter the decision-making function. This is driven by several factors:
- Transparency: Industry surveys have found that respondents view communication/transparency as one of the top considerations for hedge fund allocation decisions.
- Overfitting: With fast-changing financial markets, overfitting is often a concern, particularly for investors that do not develop the underlying models themselves.
- Lack of historical data: An estimated 90 percent of data in existence today—much of it alternative data—was created in the past two years; without crisis-relevant data, ML might not behave in a suitable way if another Great Recession were to happen. Indeed, many researchers cannot wait for full validation, as it could require decades of data to develop confidence that an algorithm is robust in a statistical sense. Several factors, such as regime shifts, higher-order interactions, and changing sources of data mean that there can be a leap of faith for researchers leveraging these methods.
- Algorithm aversion: People tend to prefer human forecasters over statistical algorithms, and more quickly lose confidence in algorithms than human forecasters after seeing them make the same mistake. Interestingly, giving individuals some control of the algorithm’s forecasts—even if extremely restricted—helps people overcome this “algorithm aversion”.
- Mismatch of talent and needs: As quantitative methods have evolved quickly, many institutional investors may not have the necessary talent in-house to build or maintain ML models.
Case study
State Street Global Advisors has introduced three exchange-traded funds (ETFs) built with proprietary index methodologies developed by Kensho Technologies. The ETFs track companies that provide AI-relevant products and services; natural language processing (NLP) is used to parse through regulatory filings to understand company exposure to AI technologies.
Matthew Bartolini, head of SPDR Americas research at State Street Global Advisors, said: “The indices are constructed themselves using the very advanced technologies that the ETFs are trying to invest in.”
He added: “The ETFs are built around the fact that algorithms can dissect information in a very systematic way, processing data in 45 seconds that would take a human a full day to understand. In this case, AI is doing exactly what an investor would expect an intelligent human would do—while being highly efficient.”
Opportunities in ML for quant-driven hedge funds
Equities-focused hedge fund usage of ML has been substantial. One estimate is that pure ML players have about $10 billion in assets under management; this number would not include “commingled” strategies, in which ML methods are used to inform trades but there is still some discretion involved. Some drivers include:
- Increased hedge fund investor comfort with algorithms: Multiple quant funds use AI-based models. For some of these funds, assets have grown rapidly as investors have flocked to algorithmic traders.
- High barriers to entry: Many asset managers are not willing to enter the ML space, posing an opportunity for those willing to make the investment. Multiple industry surveys have found that the toughest obstacles that financial executives see in introducing ML technology are the cost of systems and adoption, shortage of relevant specialised skills, and senior management buy-in. State Street’s h head of trading and algorithmic strategies, Nick Delikaris said: “There are still a lot of barriers to the adoption of ML and other newer quantitative methods, including lack of transparency, expensive hardware and data needs, and the shortage of talent. Those willing to make the investment may benefit from opportunities others are not seeing from more conventional techniques.”
Outlook for ML in beyond equities
In this piece, we have focused on ML in equity markets. While equity managers have taken some time to embrace ML technologies, it appears that interest is nearing the steep part of the curve, with usage expanding fairly rapidly. Looking at other parts of finance, we see multiple opportunities for ML:
- Research: Rajeev Bhargava, head of investor behaviour research at State Street Associates, noted the growth in alternative data and ML in the financial research space. Bhargava explained: “There has been a massive increase in interest in leveraging alt data in financial research. Driven by this interest and increased computing power, alternative data will become increasingly accessible to the masses.”
- Treasuries: Despite the fairly mature nature of the market, there may be room for investors to leverage ML to shape their trading strategies. For example, some studies have found that off-the-shelf ML programmes were a value-add in predictive 10-year treasuries strategies, which is pretty significant given that market is mature and most gains are viewed to have been arbitraged away.
- Corporate debt: Unlike in equity trading, where electronic trading is nearly universal, most corporate debt transactions are still carried out via phone or messaging. There have been some steps toward trying algorithmic trading in corporate debt, with UBS testing a computer programme to price and trade bonds as of early last year. ING has also taken a step toward adopting ML, with its adoption of AI-driven bond trading tool Katana. Going forward, we anticipate that ML will be increasingly adopted in the corporate bond space as the entire space moves toward increased implementation of electronic trading.
As with all new technologies, the adoption curve for ML may be steep. As storage becomes cheaper, calculations become faster (for example, via graphics processing units, field programmable gate arrays, quantum computing, and so on), and data sources become increasingly diverse—and as ML becomes part of a standard academic curriculum—we anticipate that ML will become more commonplace in financial analysis. We are already starting to see more widespread adoption of ML in equity trading, and anticipate other financial markets—such as corporate bonds and treasuries—to follow. Until that future state, early adopters may benefit from the lack of widespread adoption to find alpha in differentiated strategies.
Figure one: Potential use case of machine learning equity inputs/factors, by group

NO FEE, NO RISK
100% ON RETURNS If you invest in only one securities finance news source this year, make sure it is your free subscription to Securities Finance Times
100% ON RETURNS If you invest in only one securities finance news source this year, make sure it is your free subscription to Securities Finance Times
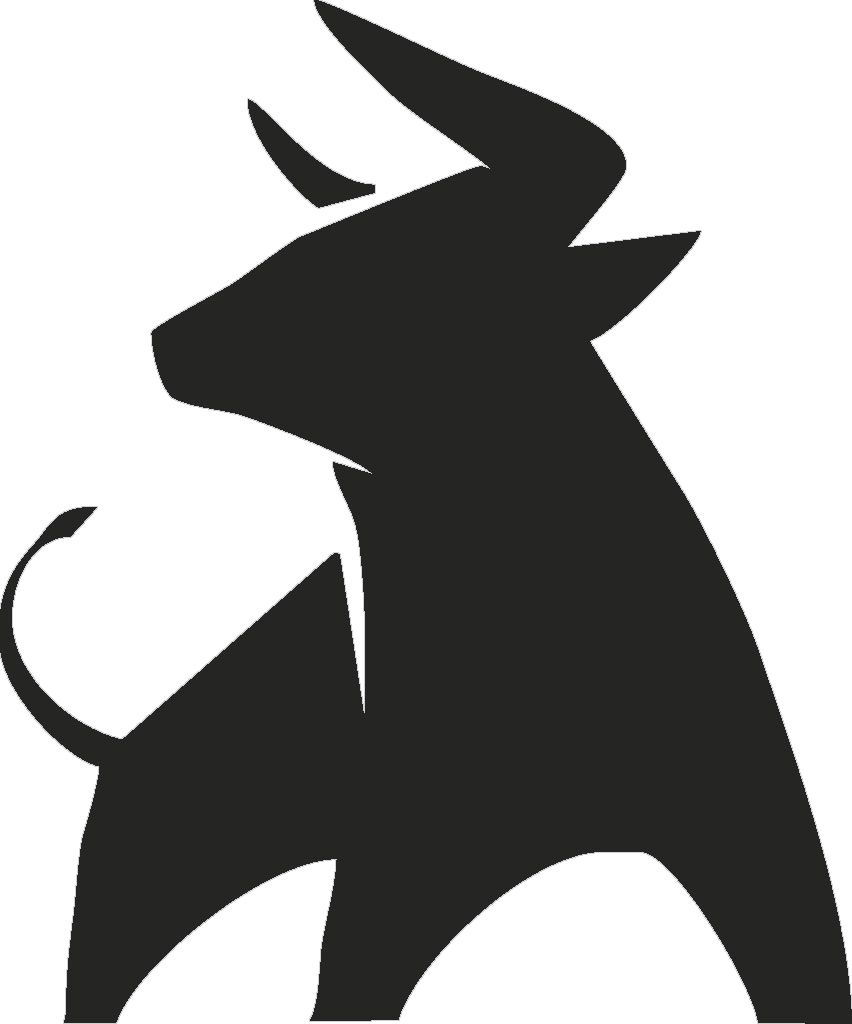